Working in Biodata and developing Labguru over the years allowed me to meet scientists from many companies and exposed me to the challenges scientists in pharmaceutical and academic labs face in their day-to-day work.
One of the things I came across is the need to structure data in order to have the ability to analyze and defend results. Talking to scientists it appears that reviewing, arranging, and going over results performed by lab technicians and students, is almost an impossible mission when vast amounts of data are scattered in various spreadsheets and not in a structured form. There is a great need for good solutions to structure unstructured scientific data.
Structured Vs. Unstructured Data
Structured data enables scientists to collect data in a methodical form with a precise set of requirements. When doing so, it is forcing the experiment performer to have certain information data objects, which in turn will need to have certain properties (i/e data types).
Unstructured data includes text files (spreadsheets, emails, word files, etc.) and machine-related data. Data streams from various instruments and analysis systems need to be unified into one final data silo that will enable comparison results from different experiments. Analyzing unstructured data at this time is still a challenge as there is no internal structure. Using traditional analyzing tools to analyze unstructured data is cumbersome and time-consuming. In addition, it is hard to identify patterns across an experiment and integrate data from multiple sources. When the outcome is structured in advance the ability to analyze the results is much easier and more productive, eliminating the margin of error and the need to search for the results, regardless of who performed the experiment.
Personnel costs when working with unstructured data is an additional pain point. The absence of structure creates inefficiency, and time loss as much time and human resources are wasted on documenting data and analyzing results without the ability to see the big picture, spending too much time on separating the wheat from the chaff.
The advantage of structured data, created in an enforced unified format, is clear. With the amount of data generated, a workable unified form that enables analysis, insights, and decisions is the most practical and efficient way to use the data.
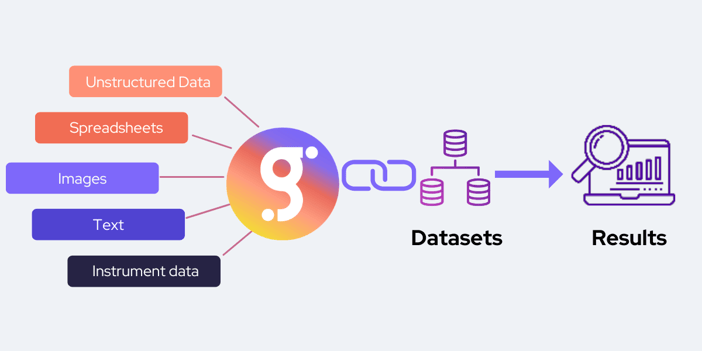
Structure unstructured Scientific data
Maintaining Data Integrity
As discussed in one of our previous blog posts, one of the major concerns of biopharmaceutical R&D executives is maintaining data integrity throughout the entire drug discovery lifecycle. Complying with data integrity regulations is a must for any industrial laboratory and is crucial for shortening drugs' time to market and avoiding unexpected expenses and delays due to regulatory violations.
Data integrity refers to the completeness, consistency, and accuracy of data. The data should be attributable, legible, contemporaneously recorded, original or a true copy, and accurate (ALCOA). Keeping your data structured can help avoid data integrity violations.
Structuring process
The challenge is creating order and structure in work procedures. Taking the time to plan and build form-like protocols will help you control the input from experiments and create order and efficiency in your lab, saving you time and money.
In all experiments, regardless of who is conducting them, it is imperative to set a working process, streamlining procedures, resulting in structured results recorded in the same way so later they can be easily analyzed.
Structuring data might require work in advance, but once the format is in place, your lab will gain efficiency and you will be able to save costs.
When attempting to create structured data it is important to:
- Map the experiment requirements in advance. Understand the workflow and data pipeline
- Decide on the methodology when data is combined from different sources
- Define a data structure and understand what is the end information needed for your analysis
- Allow flexibility in certain conditions
- Monitor errors in advance and create status reports
- Understand and model all your data sets in advance
- Link between your designed form to the relevant dataset
Using the Form Element feature in Labguru ELN to structure data
In order to help you work in an organized and efficient manner, we developed the Form Element feature. Form Element allows you to design a uniform or structured protocol, adjusted to company-specific needs and the results you are looking for, For example, QC or production procedures.
With Form Element, you can create a template that can be filled out with checkboxes, text boxes, date pickers, and more.
Forms must be designed at the protocol level and cannot be edited while used in experiments besides the data entry fields.
Form Element allows you to:
- Design the steps and the input required and lock it so work in predefined boundaries
- Set clear working procedures that need to be followed
- Design fields according to your needs
- Pre-define what is an out-of-range result and save you time later when analyzing
- Get alerts when fields were not filled out properly
- Control “Sign experiments” abilities to ensure full data capturing
- Shorten data transition to analysis by linking the form data to the relevant dataset
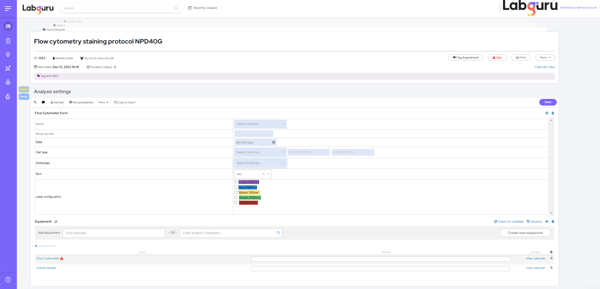
Form Element Feature- Labguru ELN
Analyze Using Datasets
Developing a carefully designed automated data pipeline will allow the consolidation of data from multiple data sources and provide your lab with reliable well-structured datasets for analytics.
The main purpose of the ‘Datasets’ is to connect the results of your experiments to the actual samples (items) that were used and get all the data into one format so it will be easier to see and analyze it. Datasets also allow you to perform searches based on the data and group together desired results from different origins to assist you with analysis.
A ‘Dataset’ in Labguru is a set of experimental results by which you can run an analysis and establish a theory on. Furthermore, files in the form of Excel or text that contain raw data and are attached to an experiment page can be turned into datasets. Labguru will then connect the data in the file and the items that exist in your inventory.
.png?width=600&height=383&name=image%20(42).png)
Labguru ELN – Dataset feature
Structuring work procedures in your lab by predefining and uniforming experiments so data outputs can be easier analyzed will save you time and money, resulting in a more efficient lab, help you maintain data integrity, and comply with regulations.
To learn more about how Labguru ELN will help you be more efficient, book a demo below!
Read more blog posts: